Having previously explored the FutureHouse Platform’s agents in tasks such as identifying tailor-made laws and generating a literature review on legislative backsliding, we now directly compare its Falcon agent and OpenAI’s o3. Our aim was to assess their performance on a focused literature search task: compiling a ranked list of the ten most relevant peer-reviewed journal articles on machine coding for the Comparative Agendas Project. Our test revealed that Falcon currently suffers from significant limitations: although the agent indicates that it draws on OpenAI’s models — including GPT-4.1 and o3 — its output was inconsistent and poorly curated. In stark contrast, OpenAI’s o3 model, accessed directly via the ChatGPT interface, generated a near-perfect result in just a few minutes: a clean, accurate, and well-structured list of peer-reviewed articles aligned precisely with the prompt.
Prompt
The objective of this task was to retrieve and rank the ten most relevant peer-reviewed journal articles on machine coding in the context of the Comparative Agendas Project. We specified clear constraints: only journal articles were to be included (excluding conference papers, book chapters, and reports), and each entry needed to list authors, title, journal name, and Scimago quartile ranking. The focus was deliberately narrow — prioritising studies that applied machine learning, automated text classification, or NLP techniques to Comparative Agendas Project data or similar policy agenda frameworks.
Conduct a deep literature search on the topic “machine coding for the Comparative Agendas Project”, and compile a ranked list of the top 10 peer-reviewed journal articles related to this topic.
Constraints:
- Only include peer-reviewed journal articles (exclude conference papers, book chapters, and reports).
- Present the results as a numbered list.
For each article, include:
- Authors
- Title
- Journal name
- Scimago Journal Rank (quartile: Q1–Q4)
Focus:
Prioritise publications that directly involve machine coding, automated text classification, or NLP techniques applied to Comparative Agendas Project data or similar policy agenda research.
Falcon’s Performance: Incomplete Filtering, Misclassified Sources, and Ranking Inconsistencies
Falcon’s output fell significantly short of expectations, despite the clear constraints outlined in the prompt. Although the platform interface confirmed that the agent had used both OpenAI's o3 and GPT-4.1-mini models to generate the response, the quality of the resulting literature list was highly inconsistent. While the first few results included relevant peer-reviewed articles, the list quickly deteriorated, incorporating conference papers, unpublished theses, and unrelated studies — many of which lacked any meaningful connection to the Comparative Agendas Project. Strikingly, the agent assigned Scimago Journal Rankings to items labelled “unknown journal,” raising doubts about the internal logic of its classification process.
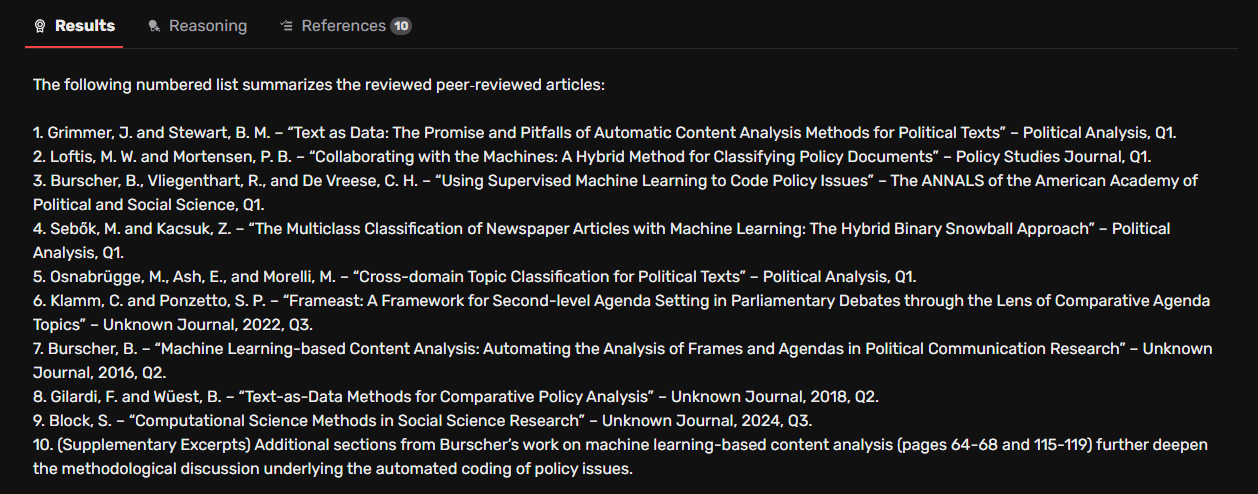
Even more puzzling, the “Reasoning” tab revealed that Falcon had retrieved additional, more suitable sources during its search but failed to include them in the final ranked list. This inconsistent filtering and ranking severely undermines the tool’s reliability for precision literature searches.
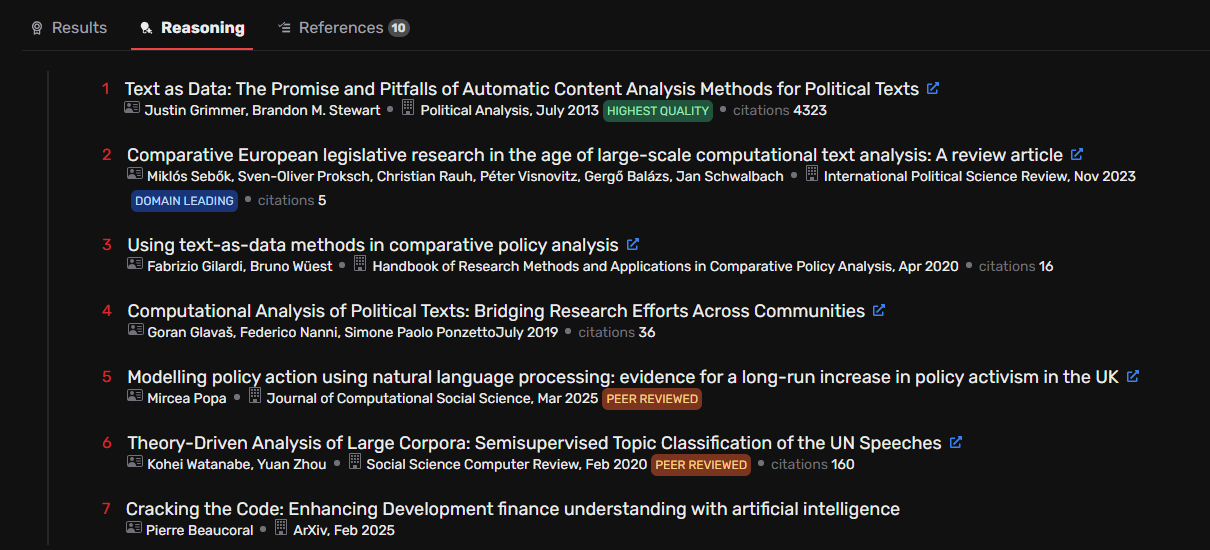
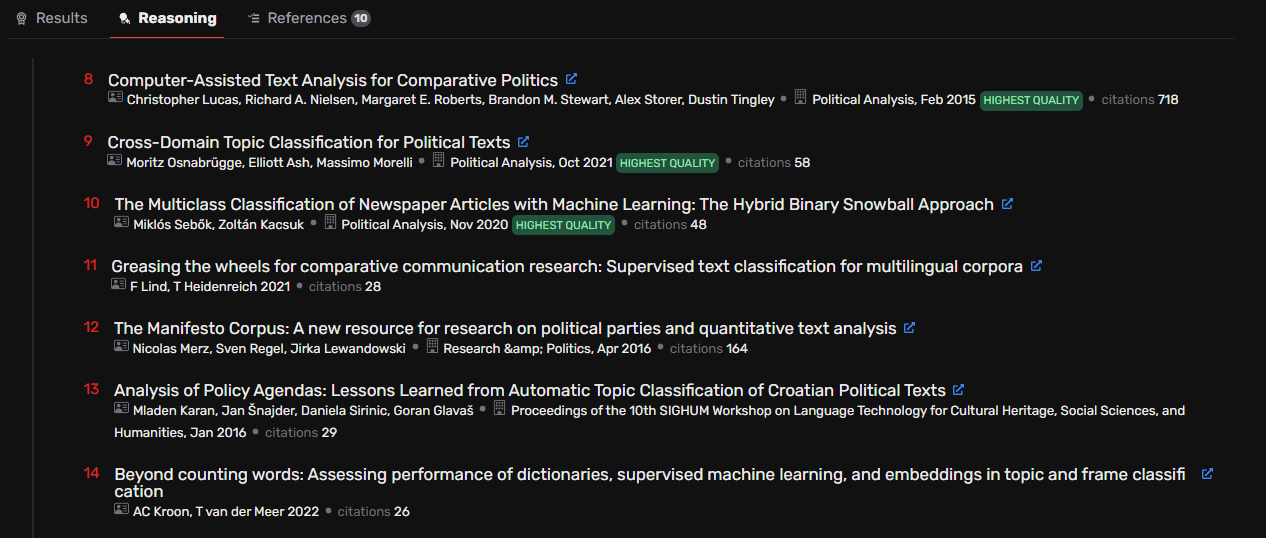
Taken together, these issues indicate that Falcon’s filtering and source evaluation mechanisms remain unreliable. The agent could not adhere to basic inclusion criteria, misclassified several sources, and failed to prioritise the most relevant papers despite having retrieved some of them. Given this performance, its usefulness for precision-focused literature retrieval remains highly limited.
OpenAI o3’s Output: Accurate, Structured, and Fully Source-Linked
In sharp contrast to Falcon, OpenAI’s o3 model — accessed through the ChatGPT interface with the same prompt — delivered a precise and well-structured result within minutes. All ten items in the returned list adhered to the given criteria: each was a peer-reviewed journal article, clearly relevant to machine coding or automated classification in the context of policy agenda research, and directly connected to or inspired by the Comparative Agendas Project.
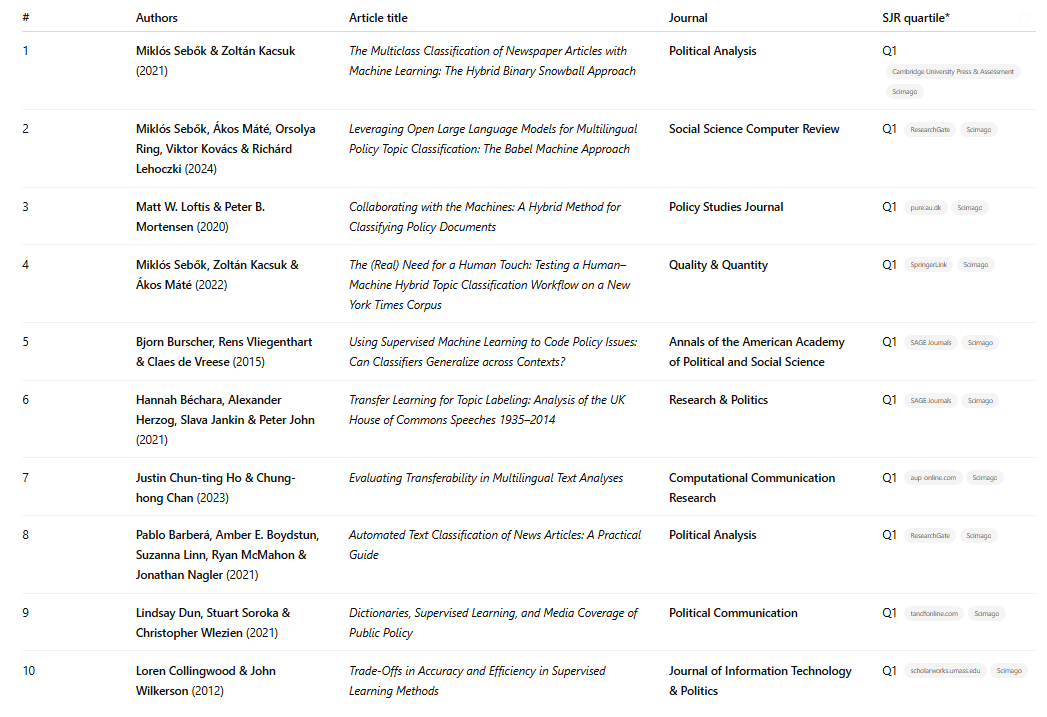
Most notably, the bibliographic details were fully accurate: author names, article titles, journal names, and publication years were correct and consistently formatted. Each journal was appropriately linked to its homepage or DOI, and the Scimago Journal Rank (Q1–Q4) was correctly cited — complete with direct links to the corresponding Scimago pages. There were no fabricated entries, nor any inclusion of conference papers or reports. In short, the model demonstrated a far better understanding of both the task constraints and the quality criteria for academic literature.
This output shows that, at least in its current implementation, OpenAI’s o3 can outperform Falcon in highly targeted literature retrieval tasks. While this may not apply to all domains or research types, for topics with a well-established scholarly footprint and clearly defined inclusion rules, o3 provides a strong foundation for conducting literature searches in academic setting.
One minor limitation did surface: for two open access article, the model linked to the author’s ResearchGate profile instead of the publisher’s official journal page, even though the article is openly accessible via the journal’s own website. While this did not affect the accuracy of the bibliographic data, it highlights the importance of verifying links and access points when using GenAI outputs for citation or dissemination.
Recommendations
Our comparison shows that the Falcon agent is currently not well-suited for high-precision literature searches on narrowly defined academic topics. Despite claiming to use OpenAI models for reasoning and answering, Falcon failed to meet key prompt constraints and included misclassified, irrelevant, or unverifiable sources in its final list. By contrast, OpenAI’s o3 model, accessed through the ChatGPT interface, generated a well-structured and fully documented top 10 list. It accurately identified relevant peer-reviewed articles, correctly cited journals, and even included direct links to Scimago rankings—demonstrating its reliability for this task.
That said, we strongly advise researchers to validate any AI-generated bibliographic output. Even high-performing models remain prone to hallucinating references, mixing up metadata, or omitting key works. Generative AI tools can significantly accelerate literature discovery, but their outputs should always be cross-checked against trusted academic databases.
The authors used OpenAI o3 [OpenAI (2025) OpenAI o3 (accessed on 19 May 2025), Large language model (LLM), available at: https://openai.com] to generate the output.