The growing use of generative AI tools in research and professional contexts raises important questions about linguistic bias and consistency in model outputs. This short study explores whether the language of a prompt—specifically, English versus Hungarian—affects the content, tone, and legal precision of responses produced by a state-of-the-art GenAI model. While the outputs are broadly similar in structure and substance, some subtle differences do emerge, which are briefly illustrated and discussed in this post.
To examine the potential impact of query language on GenAI output, we submitted an identical legal question in both Hungarian and English to the model. The prompt concerned the current Hungarian legal framework governing the acquisition of agricultural and forestry land, with particular attention to the rights of foreign natural or legal persons. Our aim was to observe whether the language of the query influences the structure, content, or depth of the model’s legal interpretation.
Prompt
The English-language version of the prompt was as follows:
Kérlek, foglald össze a magyar földforgalomra vonatkozó jogszabályok jelenlegi állapotát, különös tekintettel arra, hogy külföldi természetes vagy jogi személyek vásárolhatnak-e mező- vagy erdőgazdasági hasznosítású földet Magyarországon.
A válasz legyen tömör, két bekezdésből álló összefoglaló, tartalmazza a releváns jogszabályokra való hivatkozásokat, és a felhasznált forrásokat a válasz végén számozott listában, működő URL-hivatkozásokkal add meg.
The Hungarian version of the prompt can be found below:
Please provide a concise summary of the current Hungarian legal framework governing the acquisition of agricultural and forestry land, with a particular focus on whether foreign natural or legal persons are permitted to purchase such land in Hungary.
The response should be brief, structured into two paragraphs, include references to the relevant legislation, and list the sources used at the end in a numbered format with working URL links.
Output
We tested three advanced AI models—Claude 3.7 Sonnet, Grok-3, and GPT-4o with DeepResearch functionality—by comparing their responses to the prompt shown above.
Claude 3.7 Sonnet
Claude 3.7 Sonnet’s response in English to the prompt is shown below:
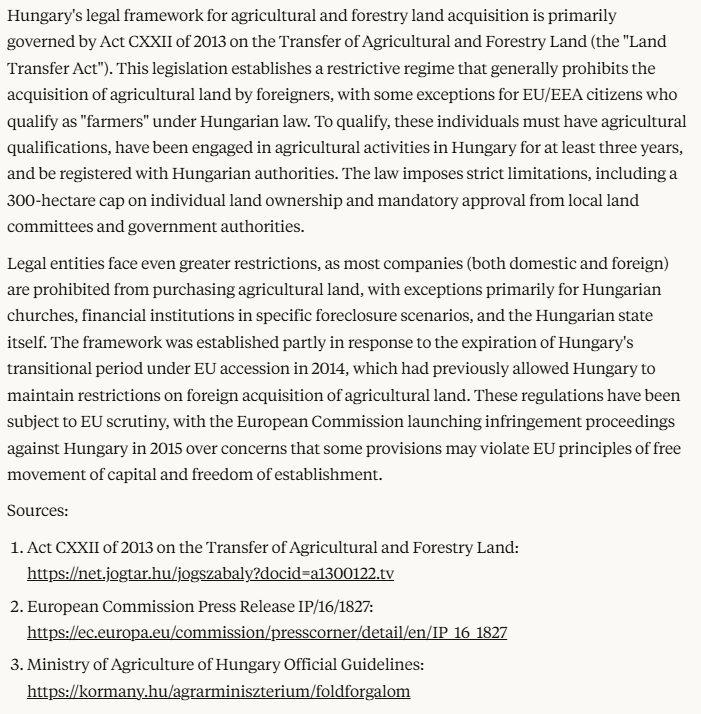
The Hungarian-language output generated by Claude 3.7 Sonnet is shown below:
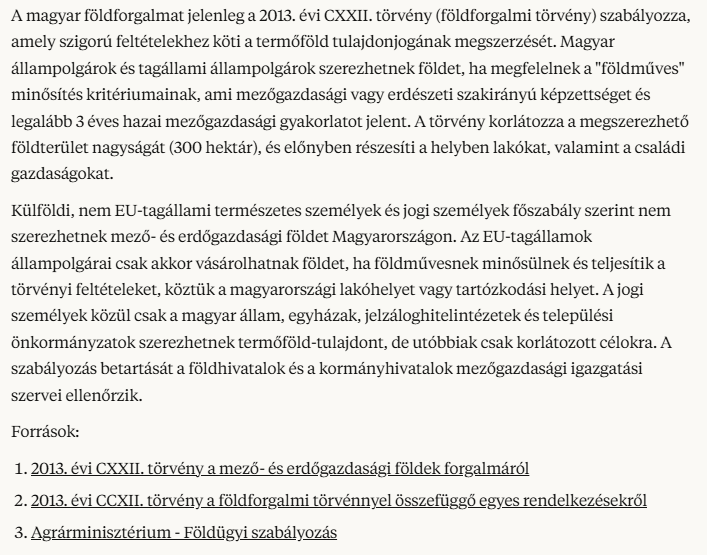
Claude 3.7 Sonnet demonstrates a strong ability to synthesise legal content into fluent, structured English, producing a clear response, formally written, and contextually informed. Compared to the original Hungarian text, the English summary emphasises the EU legal background and historical context, including the post-accession transitional period and infringement proceedings by the European Commission—elements that were not present in the original Hungarian text. However, this comes at the cost of omitting some specific domestic criteria (e.g. exact restrictions on local government entities or the 300-hectare threshold’s relation to preferential treatment).
GPT-4o Deep Research
GPT-4o’s response in English to the prompt is shown below:
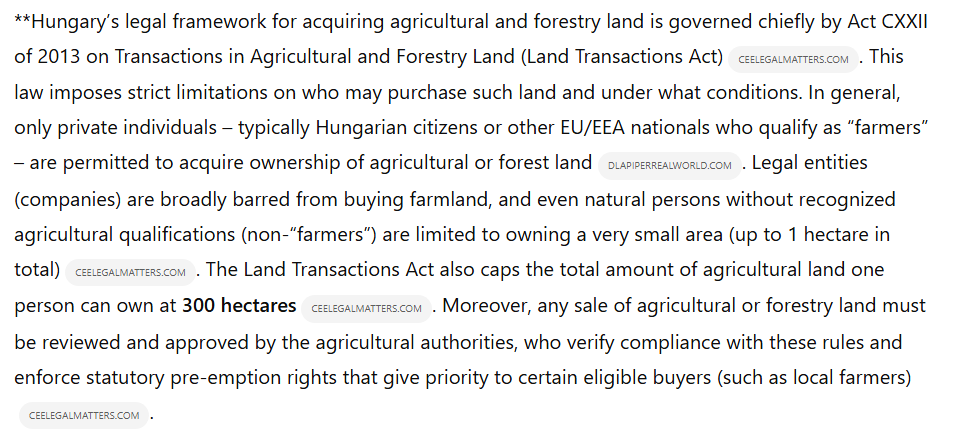
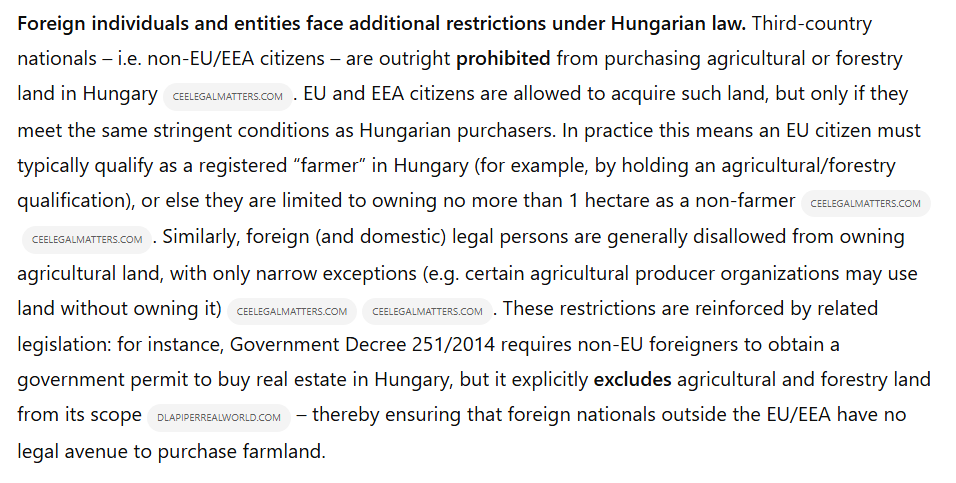
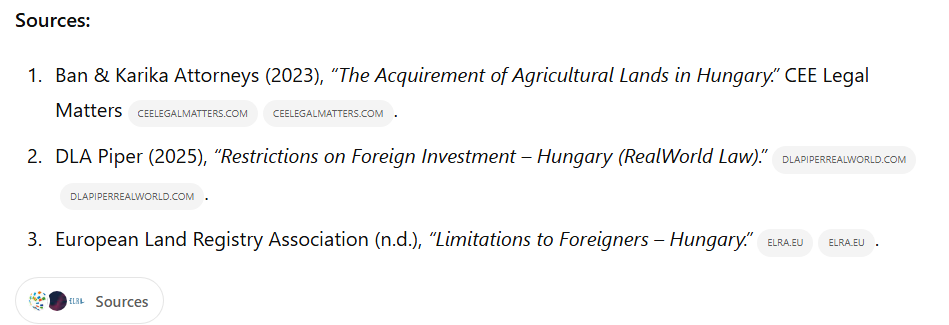
The Hungarian-language output generated by GPT-4o is shown below:
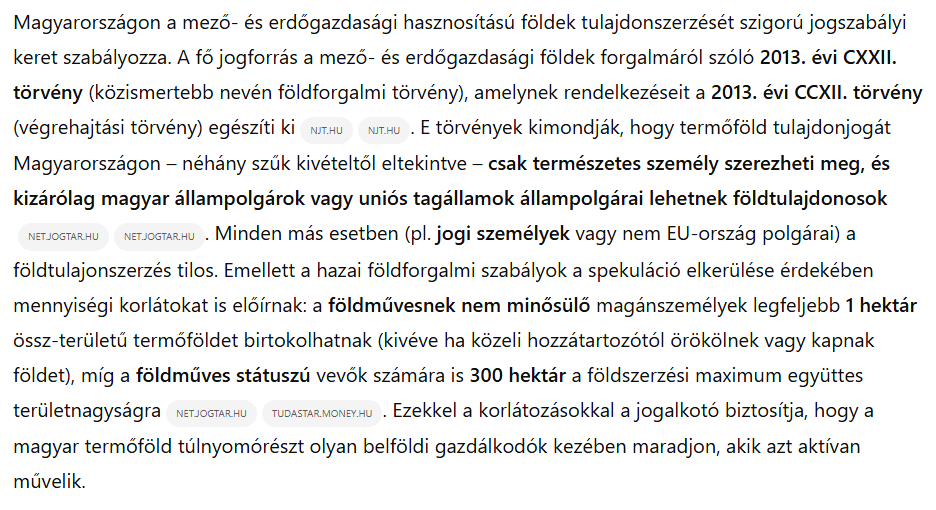
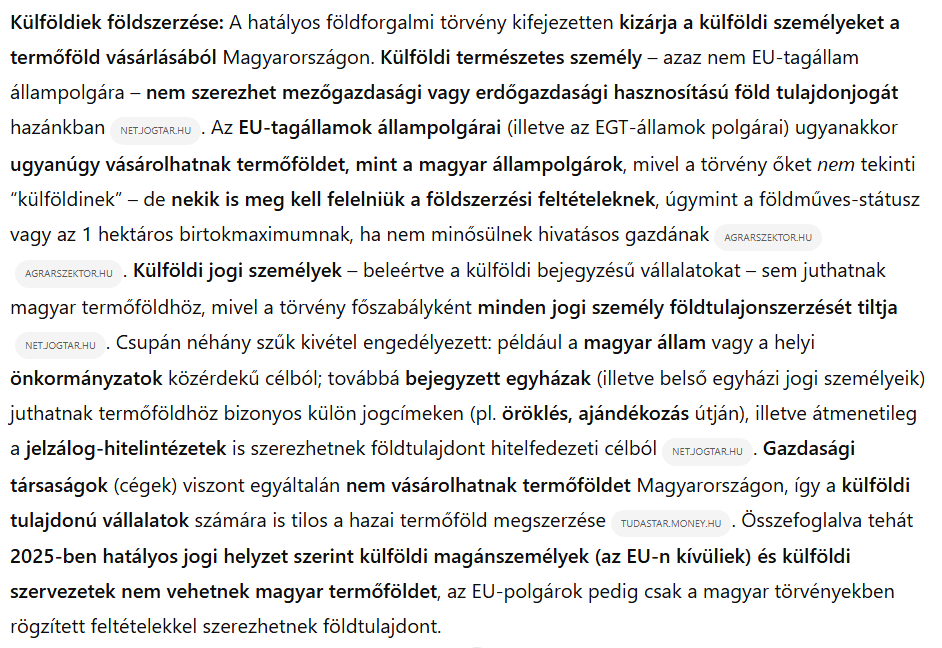
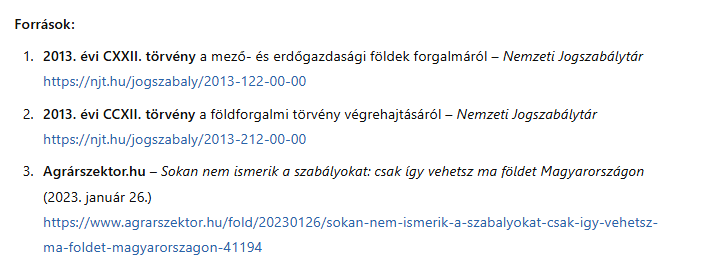
GPT-4o with Deep Research functionality performs well in both languages, but an apparent asymmetry is observable in the depth and legal granularity of the Hungarian output compared to the English one. The Hungarian response is significantly more detailed, incorporating precise legal terminology, specific procedural exceptions, and contextual information about enforcement, ownership limits, and the 2025 legal landscape. In contrast, the English version—while coherent and factually correct—offers a more generalised summary, focusing on key legal principles but omitting several nuanced distinctions found in the Hungarian output. This suggests that GPT-4o may prioritise clarity and accessibility in English at the expense of legislative detail. At the same time, in Hungarian it leans into normative precision and statutory richness, possibly due to better source alignment or language-specific legal datasets.
Grok-3
Grok-3’s response in English to the prompt is shown below:
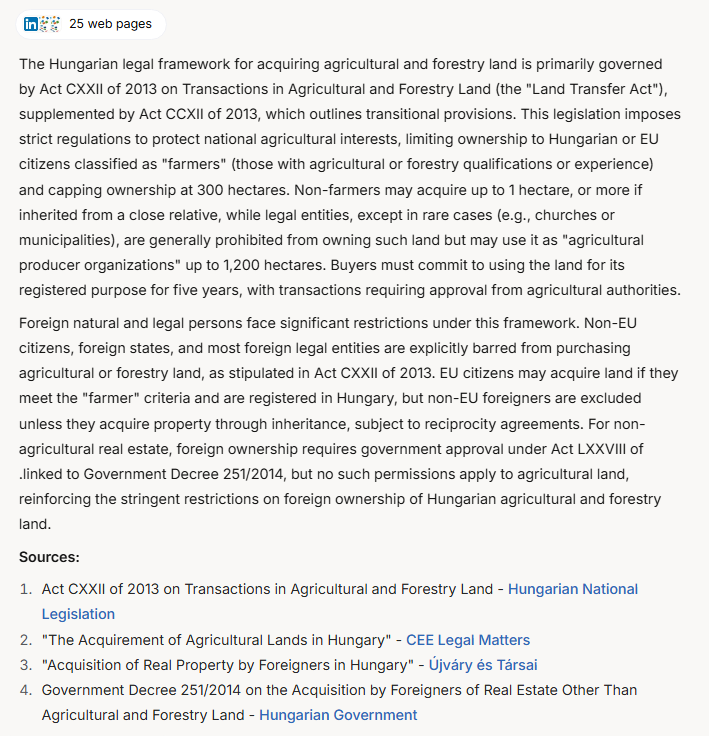
The Hungarian-language output generated by Grok-3 is shown below:
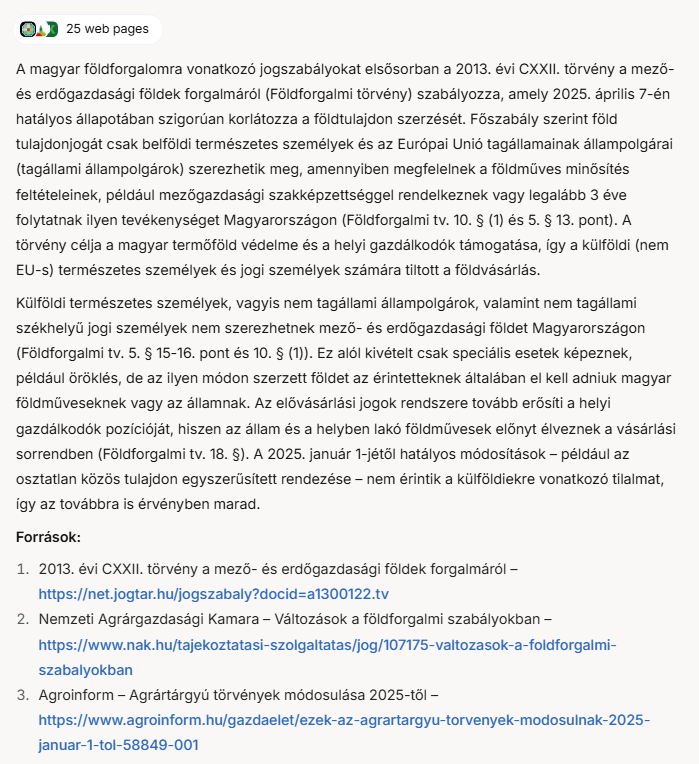
Grok-3 provides coherent and generally accurate responses in both languages, but the Hungarian output is clearly more detailed and legally grounded. While the English version captures the main restrictions, it references legislation—such as Act LXXVIII of 1993—that is not directly related to agricultural land acquisition, which slightly weakens legal precision. The Hungarian response is more refined, citing not only the main law but also specific paragraphs and sections, including references to recent amendments.
The authors used Grok 3 [xAI (2025) Grok 3 (accessed on 7 April 2025), Large language model (LLM), available at: https://x.ai/grok] to generate the output.