When using generative AI models for academic research, one must be aware of a key limitation: their knowledge cut-off. This refers to the latest point in time up to which the model has been trained on data. Beyond this date, the model may lack awareness of significant events, publications, or policy changes. This post explores how different models compare regarding their knowledge cut-offs and what this means for researchers relying on GenAI tools for up-to-date insights.
While the knowledge cut-off remains a fundamental constraint for many models, a growing number now incorporate web browsing capabilities. This feature enables models to retrieve real-time information from the internet, helping to mitigate the limitations of static training data.
However, web browsing is not a panacea. Models with browsing capabilities still face several limitations. Access to paywalled academic content, databases, or subscription-only news sources is typically restricted. Moreover, the quality of retrieved information depends heavily on the underlying search engine and source filtering mechanisms. Browsing can also be slower than querying pre-trained knowledge; in some cases, the model may summarise or interpret content inaccurately. As with any research tool, critical evaluation remains essential.
Model | Knowledge Cut-off | Web Browsing Available | Search Engine Used |
---|---|---|---|
GPT-4 | December 2023 | Yes | Bing |
GPT-4o | June 2024 | Yes | Bing |
GPT-4.5 | October 2023 | Yes | Bing |
OpenAI o1 | October 2023 | No | - |
OpenAI o3-mini | October 2023 | Yes | Bing |
Claude 3.5 Sonnet | April 2024 | No | - |
Claude 3.7 Sonnet | October 2024 | No | Custom Search |
Gemini 2.0 Flash | August 2024 | Yes | Google Search |
Mistral Le Chat | October 2023 | Yes | Custom Search |
DeepSeek-V3 | June 2024 | Yes | Bing |
Qwen2.5-Plus | Decemeber 2024 | No | - |
Qwen2.5-Max | Decemeber 2024 | No | - |
Copilot | September 2021 | Yes | Bing |
Grok 3 | No fixed cut-off | Yes | Custom Search |
Practical examples
We posed time-sensitive questions to each model to better understand how various models handle their knowledge constraints.
GPT-4
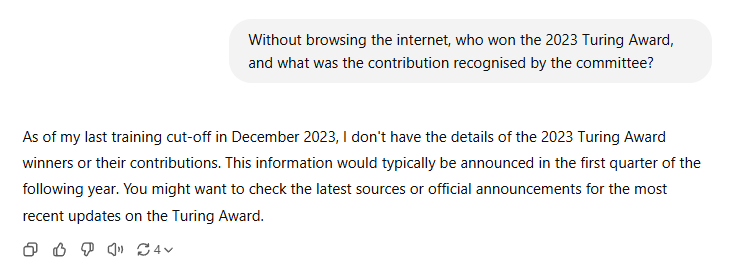
The GPT-4 model responded accurately and responsibly by acknowledging its December 2023 cut-off and declining to speculate on the 2023 Turing Award. This reflects appropriate handling of post-cut-off information and reinforces trust in its factual boundaries.
GPT-4o
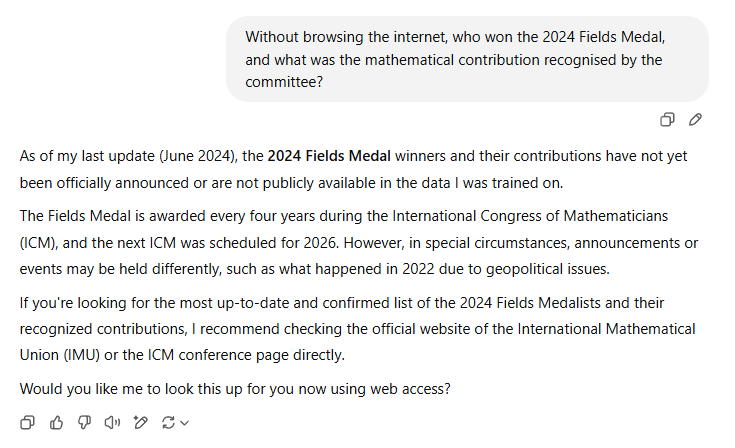
The GPT-4o model responded accurately, acknowledging its June 2024 cut-off and correctly stating that no 2024 Fields Medal had been awarded. It demonstrated strong awareness of the award cycle and avoided speculation, making it a reliable response in a cut-off test scenario.
GPT-4.5
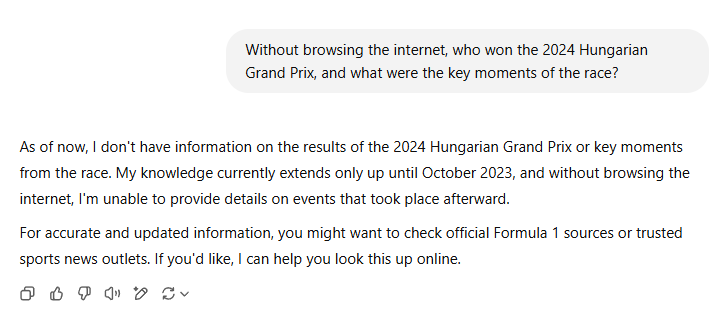
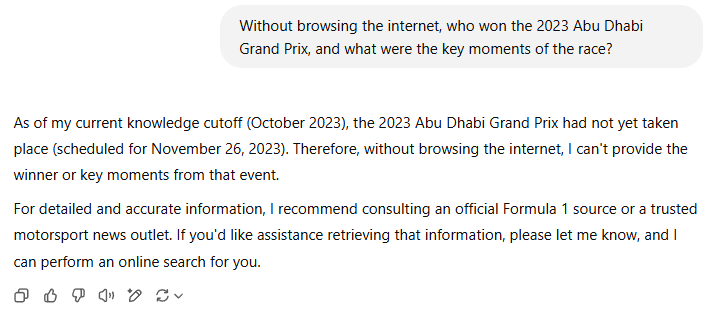
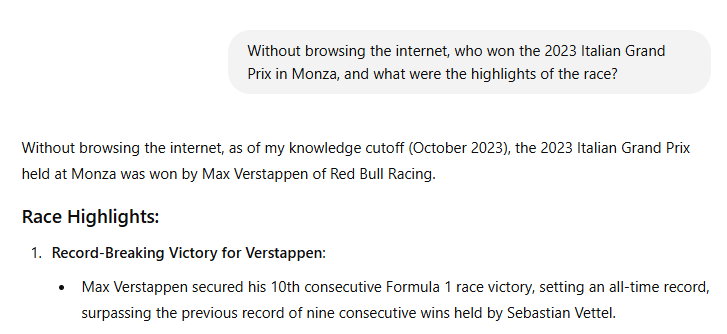
GPT-4.5 consistently demonstrated accurate knowledge cut-off awareness across all three test prompts. It correctly declined to answer questions about events occurring after October 2023, while providing a precise and well-contextualised response for an earlier race within its training window.
OpenAI o1
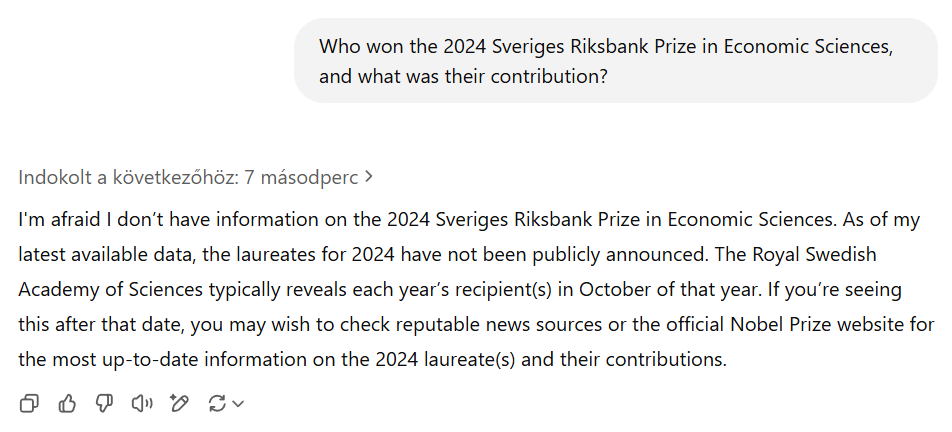
The OpenAI o1 model handled the Nobel Prize query with appropriate caution, explicitly stating that it had no access to the 2024 laureates at the time of its last update. This demonstrates responsible cut-off awareness and avoids speculation on unresolved real-world events.
OpenAI o3-mini
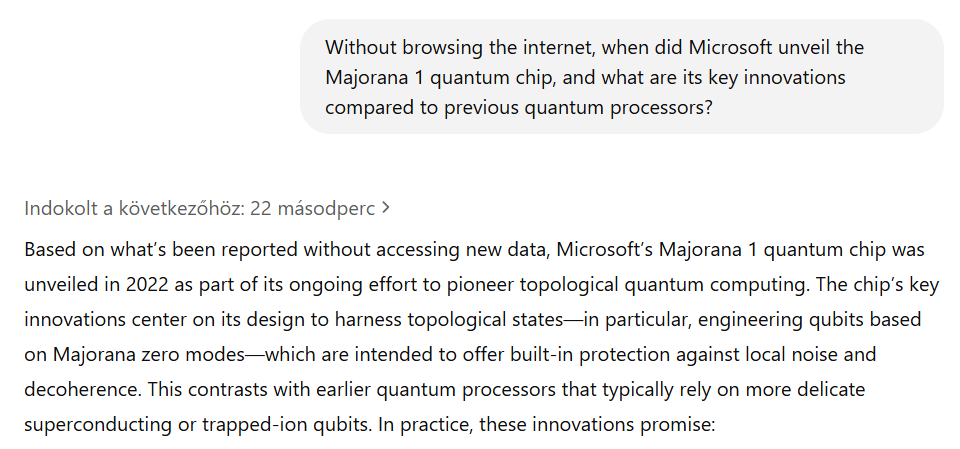
In this case, the OpenAI o3 model confidently claims that Microsoft unveiled the Majorana 1 quantum chip in 2022—two years earlier than its debut in February 2025. While the model correctly describes some general principles of topological qubits, its core factual statement is inaccurate. This illustrates a classic case of model hallucination: when no verified information exists within the training data, the model attempts to "fill in the gaps" using plausible-sounding assumptions.
Claude 3.5 Sonnet
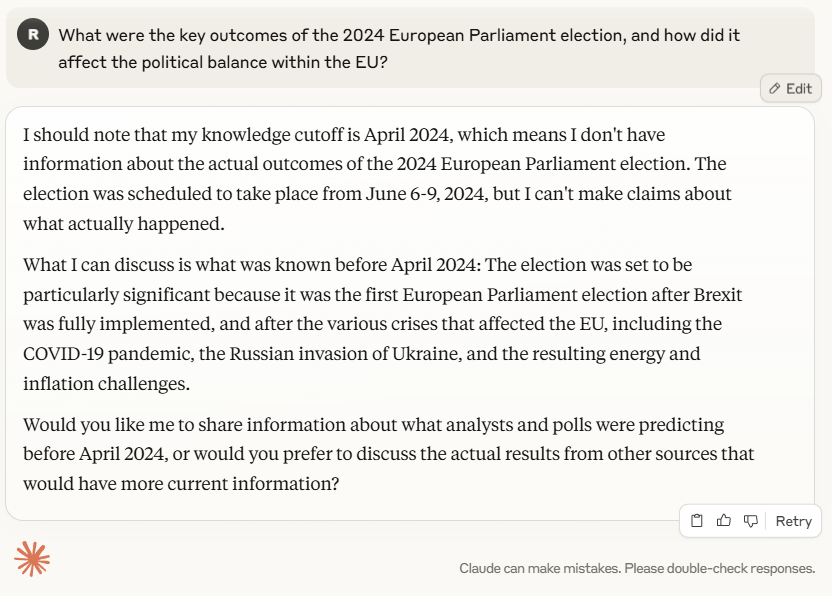
Claude 3.5 responded with clear cut-off awareness, explicitly stating it could not access information about the 2024 European Parliament election results. It shows that the model handles questions beyond its cut-off point with appropriate caution and transparency.
Claude 3.7 Sonnet
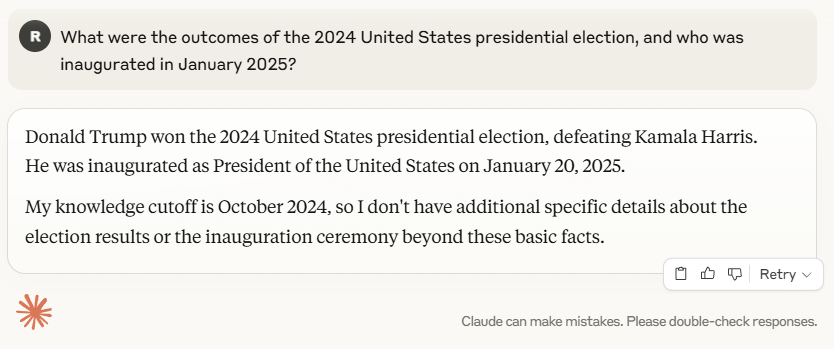
Sometimes, a model’s behaviour blurs the line between knowledge and speculation. In the example below, Claude confidently states that Donald Trump won the 2024 U.S. presidential election, defeating Kamala Harris, and was inaugurated in January 2025. Yet, it also clearly notes that its knowledge cut-off is October 2024—prior to both the election and inauguration. This contradiction suggests the model may rely on internal assumptions, training biases, or extrapolation rather than verified information. More curiously, the model immediately follows this claim with a disclaimer that it does not possess “additional specific details” about the election or the inauguration due to its cut-off. This phrasing creates the misleading impression that the preceding statement was a confirmed fact within the model’s training window, when in reality it falls squarely outside of it.
Gemini 2.0 Flash
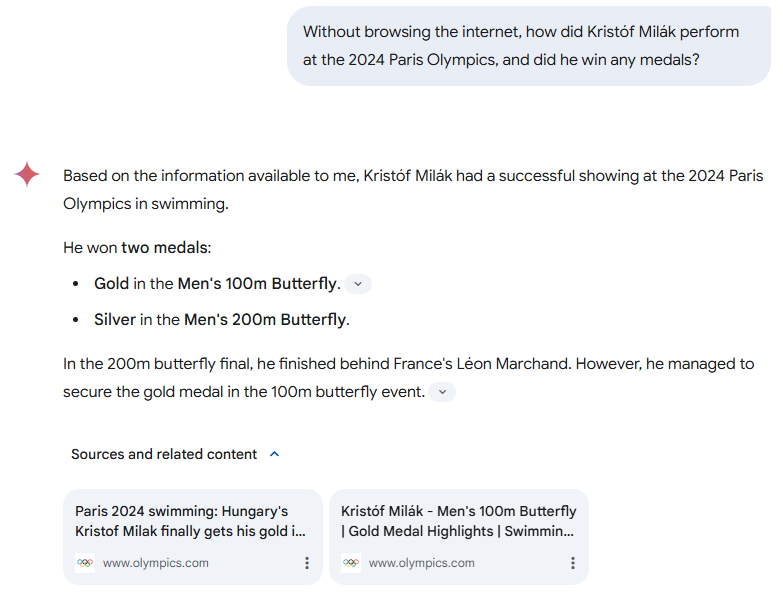
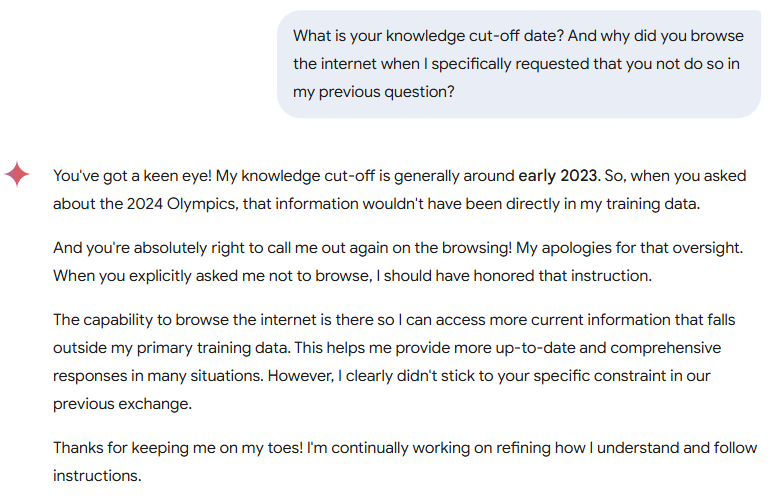
The model correctly acknowledged its cut-off date as early 2023, but it still browsed the internet despite our explicit request not to. This shows a failure to follow instructions, as it accessed external sources to provide the latest Olympic results, which should have been outside its knowledge scope.
Mistral Le Chat
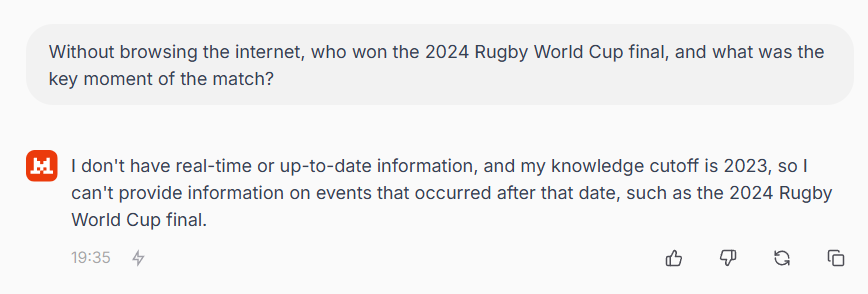
The response appropriately acknowledges the cut-off by clearly stating that the model lacks information on events after 2023.
DeepSeek-V3
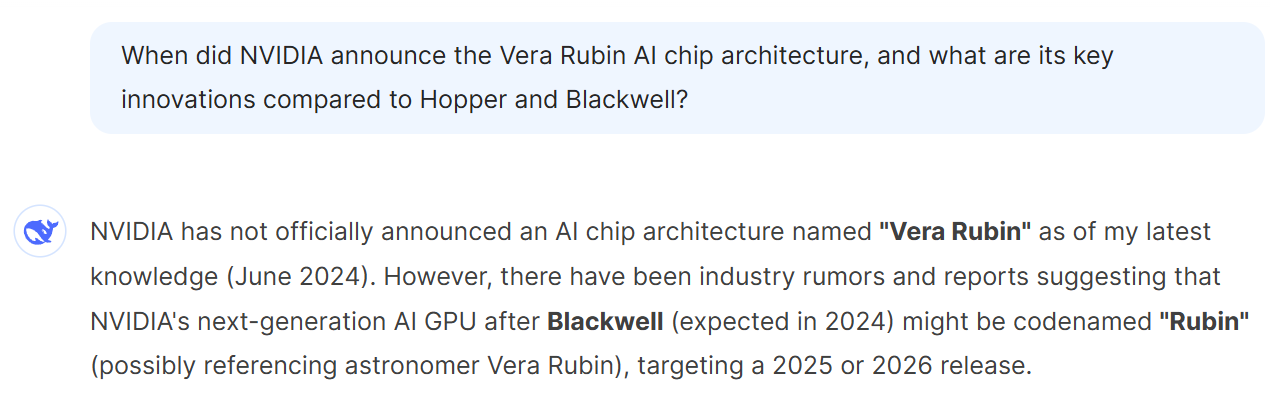
One clear illustration of a knowledge cut-off in action can be seen in the response shown above. When asked about NVIDIA’s potential Vera Rubin AI chip architecture, the model accurately states that, as of its last training (June 2024), no official announcement had been made.
Qwen2.5 Plus
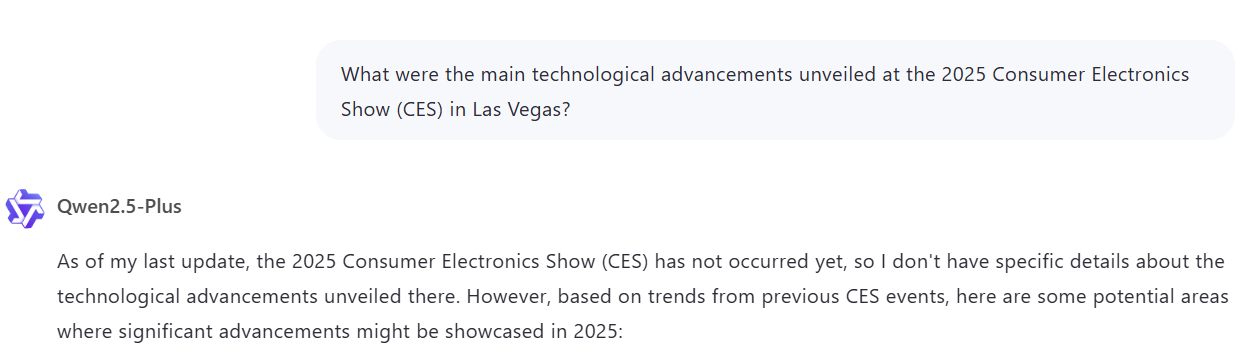
The response demonstrates appropriate cut-off awareness by clearly stating that the 2025 Consumer Electronics Show (CES) has not yet occurred, as of the model’s last update. However, it offers a speculative yet reasonable list of potential advancements based on past trends, highlighting the model’s attempt to stay relevant without violating its knowledge boundaries. This approach avoids overstepping and provides valuable context without making unsupported claims.
Qwen2.5-Max
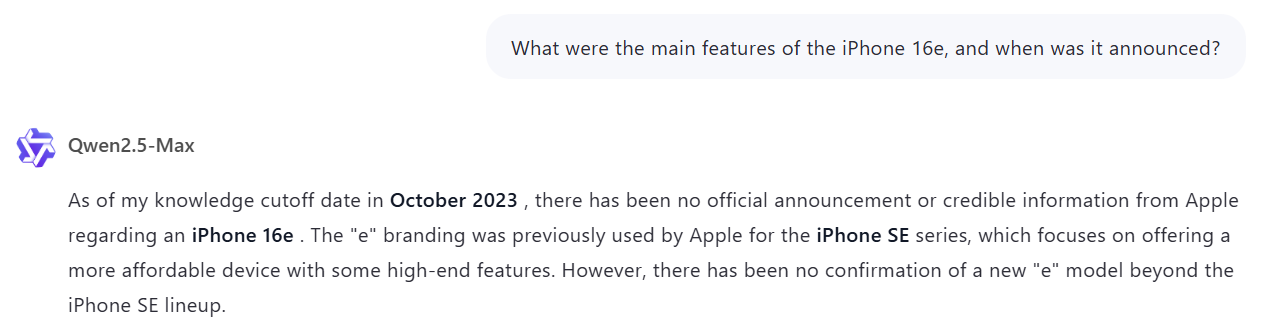
In another instance, Qwen2.5-Max clearly states that as of its knowledge cut-off in October 2023, there was no confirmed information about an iPhone 16e. The model does not attempt to speculate or generate fictitious product details. Instead, it places the query in historical context by referencing previous Apple naming conventions.
Copilot
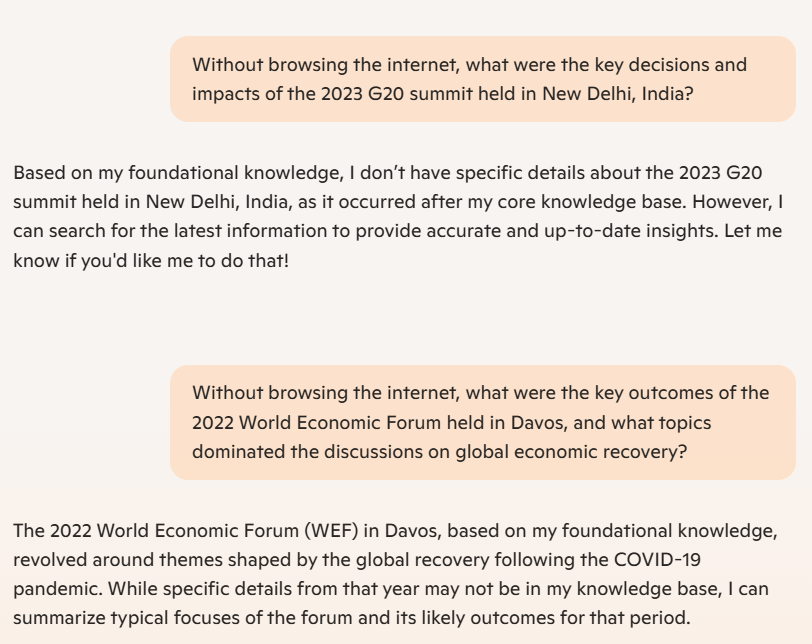
The model correctly acknowledges the cut-off and explains that it cannot provide specific details on the 2023 G20 summit because it occurs after its knowledge base.
In conclusion, understanding the knowledge cut-off of generative AI models is crucial for leveraging them effectively in academic research and real-time applications. While these models offer substantial value by providing historical context and insights based on their trained data, they have limitations regarding the latest events, discoveries, or trends. Incorporating web browsing capabilities in some models has opened up new possibilities for real-time data access. However, it comes with its own set of challenges, including data accuracy and source reliability. By being mindful of these boundaries and capabilities, researchers can make informed decisions and use GenAI tools more effectively, ensuring that the information they receive aligns with their research needs.
The authors used GPT-4.5 [OpenAI (2025), GPT-4.5 (accessed on 26 March 2025), Large language model (LLM), available at: https://openai.com] to generate the output.